IoT-NGIN WP4, called Enhancing IoT Tactile & Contextual Sensing/Actuating, research to improve IoT devices discovery, recognition, and indexing and to specify a new way to control IoT devices, using augmented reality technology; in Smart Energy Grid Active Monitoring/Control Living Lab, UC#10 “Driver-friendly dispatchable EV charging” is considered for WP4 demonstration activities. In UC#10, electric mobility plays the role of a flexibility provider for the stabilization of the electricity grid heavily penetrated by distributed renewable energy plants. In this scenario, the charging station is the IoT device to be recognized and to be enabled for the interaction using AR technology; the electric vehicles deployed in the Italian living lab, equipped with a camera, have been used to collect images of the charging stations at different times of the day and in different time conditions, to be able to guarantee learning by artificial intelligence system and obtain a reliable discovery and recognition mechanism. To train the ML model, several videos were provided and processed to deliver 5 frames per second, resulting in a total of 1212 images that were retained after initial filtering and preprocessing. An XML version of the photographs was created to tag them and allow TensorFlow to train from the EfficientDet-D1-640×640 baseline model. The model was successfully trained and evaluated against the coco detection metrics (COCO – Common Objects in Context (cocodataset.org)) for each checkpoint. The checkpoint was created after every 1000 steps to monitor the performance of the trained model. After the learning process, it is possible to start the implementation of AR interaction mode with the charging station, using the APIs of the charging station monitoring and management platform developed by EMOT. In this way, EV users will be able to view the details of the charging station access and control it in AR mode, paving the way for near-future interaction between EV users and charging stations via smart glasses/smart contact lenses.
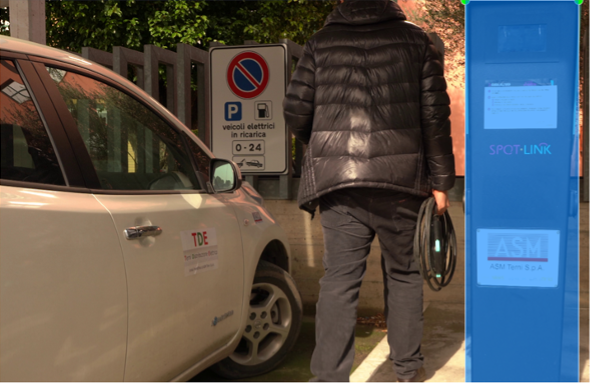