Industrial automation has seen an exponential growth in the last century. Several technological advancements have been reported and implemented in various industrial sectors to increase production efficiency by orders of magnitude and allow the handling of more sophisticated tasks. On the other hand, demands in each sector keep increasing with stricter regulations and constraints. World population is expected to exceed 9 billion people by 2050 1, 2 putting a huge burden on agriculture to feed everyone. With increasing demands and shortage of labour across the globe, agricultural technologies are starting to gain attention among farmers. Recent advancements in sensors and Artificial Intelligence technology that lets machines to train on their surrounding has made automated systems more notable. The only agricultural concept able to provide such productivity and yield increase is the concept of Precision Agriculture or Smart Agriculture with the use of state-of-the-art technologies to minimize input, maximize output and quality while maintaining low operating costs 3.
In the 21st century and the era of IoT, new technologies have been developed to be implemented in agriculture automating the whole process of crop production and crop harvesting. These technologies have the advantage of improving the agricultural yield and significantly increasing productivity. However, most of them require a huge capital investment and the farmers’ willingness to change their classical agricultural practices and adopt these new technologies. These technologies (Figure 1) 4, 5capable to implement digitally controlled farms can be generally categorised into 6:
- direct sensors monitoring the soil, plant and environmental conditions
- remote sensing and monitoring of the crops through satellite or drone images
- weeding, spraying and crop harvesting robots
- and eventually, to complete digitally controlled farming systems
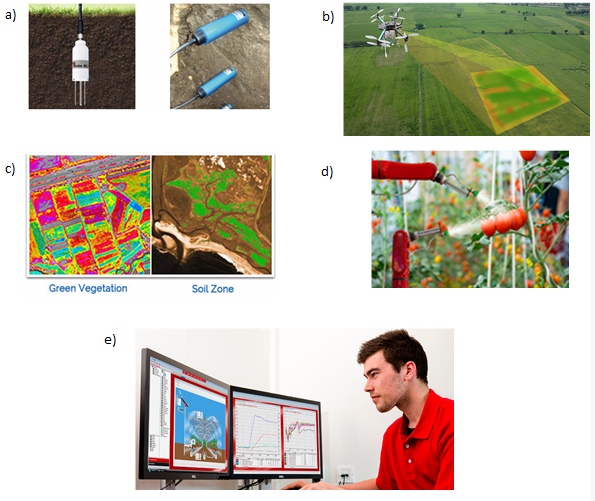
Although most of these technologies currently exist, the integration of all of them into an optimised IoT-based system has yet to be reported. Several similar IoT-based systems (e.g. Priva-maximiser [7]) have been reported but they are mostly open-loop and feature only remote control of the farms. The ability to obtain real-time data without the need for human presence, has envisioned the concept of fully automated decision-making systems for agriculture in a closed-loop feedback system. In the second decade of the 21st century, due to the continuous advancements in the field and the increasing interest by the scientific and industrial community, there are now commercially available technologies transforming classical agricultural farms into digitally controlled farms. Unfortunately, so far digitally controlled farms lack the intelligence to make unmanned decisions 8. A new concept of Automated Decision-Making System (ADMS) has been recently described in the literature that uses a combination of sensing nodes talking to each other and eventually to a central controller or PC 3. The central controller uses machine learning algorithms and artificial intelligence to make decisions on its own without the need for human control. This approach features the advantage that due to the machine learning algorithms and the use of historical data, new optimum points can be found and a new pool of knowledge can be generated without any further human input. However, the implementation of such a system has not yet been reported even today with the closest probably being Hoogendoorn iSii 4. Major challenges remain in the area of real-time and IoT compatible, sensing systems at low cost. Along with the sensor development, the electronic interfaces required to run those sensors should fulfil the strict specification requirements of the application, such as power reduction, size and cost. Communication of these sensor nodes with the central controller in high-density sensor networks without any interference, with low power and at large distances are challenges that still remain unanswered. Adding intelligence to these systems, not only through the central controller but also on-node-intelligence, along with machine learning and neural networks will highly increase agricultural productivity, improve food quality, reduce food prices and optimize the use of natural resources.
IoT-NGIN researches on enhancing Smart Agriculture with unique technological features around IoT and Artificial Intelligence. IoT-NGIN aims to achieve more effective predictions of crop diseases, combining multiple sensor measurements and plant images acquired via drones, while performing smart irrigation and precision aerial spraying, ultimately aiming increased quality of crop production and reduced use of water resources and pesticides. Moreover, IoT-NGIN develops a smart harvesting use case, powered by a mobile robot, acting as carrier able to calculate routes on the fly, detecting and avoiding any obstacles, thus ensuring human workers’ safety.
References
1N. Alexandratos and J. Bruinsma, “World Agriculture Towards 2030/2050,” 2012.
2FAO, “Global agriculture towards 2050,” 2009.
3 M. Sophocleous and J. Georgiou, “Precision agriculture: Challenges in sensors and electronics for real-time soil and plant monitoring,” 2017 IEEE Biomed. Circuits Syst. Conf., pp. 1–4, 2017.
4https://www.hoogendoorn.nl/en/product/isii-process-computer/
5https://www.cropin.com/iot-internet-of-things-applications-agriculture/
6M. Sophocleous “Towards fully automated decision-making systems for precision agriculture: Soil sensing technologies—‘The missing link” in Precision Agriculture Technologies for Food Security and Sustainability pp. 23 2021.
7https://www.priva.com/
8https://medium.com/next-generation-iot-magazine/autonomous-tractors-and-plant-level-precision-farming-how-europes-farmers-can-leverage-the-power-4b3111e25e19